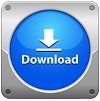

An ensemble of two state-of-the-art models reach a mean absolute error (MAE) of 0.388 eV, which corresponds to a percentage error of 13\% for an average band gap of 3.05 eV.
#Flowjo ashland download#
With this aim, a consistent dataset of 12 500 crystal structures and their corresponding DFT band gap are released, freely available for download at. In this paper, the focus is on predicting the band gap which represents one of the basic properties of a crystalline material. The complexity of the organic crystals contained within the OMDB, which have on average 82 atoms per unit cell, makes this database a challenging platform for machine learning applications. Over the past two years, the Organic Materials Database (OMDB) has hosted a growing number of calculated electronic properties of previously synthesized organic crystal structures. In summary, opt-SNE enables superior data resolution in t-SNE space and thereby more accurate data interpretation.Ībstract Machine-learning models are capable of capturing the structure–property relationship from a dataset of computationally demanding ab initio calculations. The precise calibration of early exaggeration together with opt-SNE adjustment of gradient descent learning rate dramatically improves computation time and enables high-quality visualization of large cytometry and transcriptomics datasets, overcoming limitations of analysis tools with hard-coded parameters that often produce poorly resolved or misleading maps of fluorescent and mass cytometry data. We develop opt-SNE, an automated toolkit for t-SNE parameter selection that utilizes Kullback-Leibler divergence evaluation in real time to tailor the early exaggeration and overall number of gradient descent iterations in a dataset-specific manner. A state-of-the-art algorithm for non-linear dimension reduction, t-SNE, requires multiple heuristics and fails to produce clear representations of datasets when millions of cells are projected. Accurate and comprehensive extraction of information from high-dimensional single cell datasets necessitates faithful visualizations to assess biological populations.
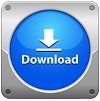